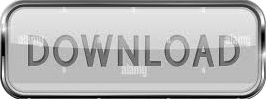
DataFrame ): raise TypeError ( "input needs to be a pandas dataframe" ) if has_intercept : if df. Args: df (pandas.DataFrame): dataframe of design matrix output from patsy has_intercept (bool): whether the first column of the dataframe is the intercept exclude_intercept (bool): exclude intercept from computation and assumed intercept is the first column default True tol (float): tolerance check to print warning if any vifs exceed this value check_only (bool): restrict return to a dictionary of vifs that exceed tol only rather than all default False Returns: dict: dictionary with keys as column names and values as vifs """ if not isinstance ( df, pd. If check_only is true it will only return a 1 if any vifs are higher than tol. Prints a warning if any vifs are >= to tol. Uses the same method as Matlab and R (diagonal elements) of the inverted correlation matrix. During the evaluation, we have noticed that APAnt also has a particular preference for hypernyms.Def vif ( df, has_intercept = True, exclude_intercept = True, tol = 5.0, check_only = False ): """ Compute variance inflation factor amongst columns of a dataframe to be used as a design matrix. This paper describes the algorithm in details and analyzes its current limitations, suggesting that extensions may be developed for discriminating antonyms not only from near-synonyms but also from other semantic relations. Evaluation shows that it outperforms three baselines in an antonym retrieval task: the vector cosine, a baseline implementing the co-occurrence hypothesis, and a random rank. The measure – previously introduced in some pilot studies – is presented here with two variants. their average rank in the mutual dependency sorted list of contexts). Such hypothesis has been implemented in APAnt, a distributional measure that evaluates the extent of the intersection among the most relevant contexts of two words (where relevance is measured as mutual dependency), and its saliency (i.e. The discriminating method is based on the hypothesis that, even though both near-synonyms and opposites are mostly distributionally similar, opposites are different from each other in at least one dimension of meaning, which can be assumed to be salient. This paper analyzes the concept of opposition and describes a fully unsupervised method for its automatic discrimination from near-synonymy in Distributional Semantic Models (DSMs). The conclusion of the last study is that extended context compresses aptness scores towards the center of the scale, raising low ratings and decreasing high ratings given to paraphrase candidates outside of extended context. I show that extended context changes human perception of metaphor aptness and that this effect is reproduced by my neural classifier.

In the final experiment of this compilation, more context is added to a sub-section of the dataset in order to study the effect of extended context on metaphor aptness rating. I then designed a deep neural network to be trained on this dataset, that is able achieve encouraging levels of performance. This dataset is annotated through crowd sourcing by an average of 20 annotators for each pair. Therefore, I could use it both for binary classification and ordering tasks. I built a dataset designed for this task, that allows a gradient scoring of various paraphrases with respect to a reference sentence, so that paraphrases are ordered according to their degree of aptness. Given a sentence containing a metaphor, the task is to find the best literal paraphrase from a set of candidates. To deal with metaphor aptness assessment, I framed the problem as a case of paraphrase identi- fication.
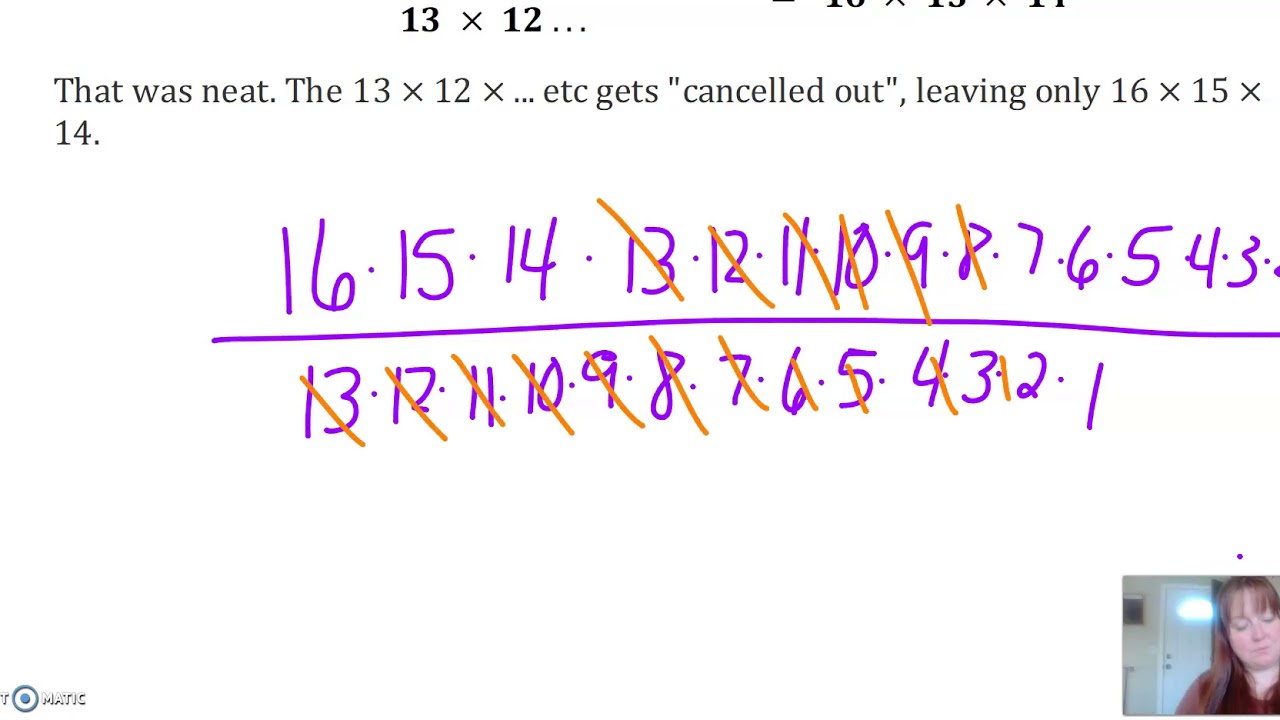
In all of the studies presented here, I have used a combination of word embeddings and neural networks. For metaphor detection I was able to use existing resources, while I created my own dataset to explore metaphor aptness assessment. I approach it as a way to assess the potentialities and limitations of our approach, before dealing with the second task. The first task has already been tackled by a number of studies. This compilation thesis provides a set of experiments to (i) automatically detect metaphors and (ii) assess a metaphor’s aptness with respect to a given literal equivalent. Secondly, the practical lack of large scale resources forces researchers to work under conditions of data scarcity. First of all, the semantic complexity of the concept of metaphor itself creates a range of theoretical complications. While it is considered an element of great interest in several branches of linguistics, such as semantics, pragmatics and stylistics, its automatic processing remains an open challenge. Metaphor is one of the most prominent, and most studied, figures of speech.
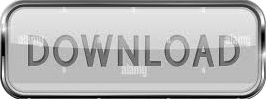